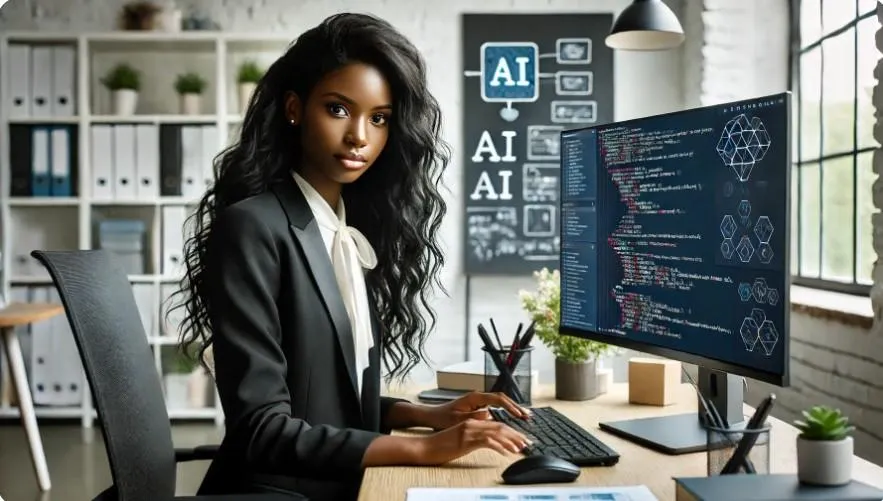
Introduction to Prompt Engineering: The Art of Guiding AI
The Primary Duties and Responsibilities of a Prompt Engineer
As the field of artificial intelligence (AI) continues to expand, the role of the prompt engineer has emerged as a pivotal one in harnessing the power of large language models (LLMs). Prompt engineers are responsible for guiding these sophisticated models, such as ChatGPT, to produce accurate and relevant outputs by crafting and refining prompts. This role requires a unique blend of technical expertise, creativity, and problem-solving skills. Let’s explore the core duties of a prompt engineer and how these responsibilities contribute to the effectiveness of AI-driven solutions.
One of the primary responsibilities of a prompt engineer is understanding the capabilities and limitations of LLMs. These models, while powerful, have specific ways in which they interpret and respond to prompts. A deep understanding of how the LLM processes language, retrieves information, and generates responses is essential. Prompt engineers must be aware of factors such as token limits, context windows, and the model's training data scope. This foundational knowledge allows them to design prompts that maximize the model’s potential while avoiding pitfalls like hallucinations, biases, or irrelevant outputs.
Crafting effective and targeted prompts is another key duty of a prompt engineer. A well-designed prompt can make the difference between an incoherent response and a precise, valuable output. Engineers must carefully word their prompts to guide the LLM toward the desired outcome, whether it’s generating content, answering complex questions, or simulating dialogues. This involves understanding not only the technical aspects of prompt design but also the nuances of the domain in which the AI is being applied. For instance, a prompt engineer working in the medical field must craft prompts that consider medical terminology and the specific needs of healthcare professionals, ensuring the LLM provides accurate and trustworthy information.
A significant part of a prompt engineer’s work involves testing and refining prompts. Prompt engineering is an iterative process that requires constant experimentation. After creating an initial prompt, the engineer must evaluate the LLM's output to determine whether it meets expectations. If the output is inaccurate or incomplete, the engineer adjusts the prompt, tests it again, and refines it until the model generates the desired response. This cycle of prompt testing and refinement is crucial for optimizing the interaction between humans and AI, ensuring that the model is as effective as possible in performing its tasks.
Finally, evaluating LLM outputs is a critical responsibility of a prompt engineer. It’s not enough to simply craft and test prompts; engineers must also assess the quality, relevance, and reliability of the AI’s responses. This evaluation requires a keen eye for detail and a strong understanding of the subject matter. Prompt engineers often work closely with subject matter experts to verify that the LLM's outputs align with real-world knowledge and standards. In some cases, engineers may need to balance the model's creativity with the need for accuracy, especially when the AI is used in fields like law, finance, or healthcare.
In conclusion, prompt engineers play a vital role in shaping the way we interact with AI models like LLMs. Their responsibilities include understanding the intricacies of the models, crafting precise prompts, refining those prompts through iterative testing, and ensuring the quality of the AI’s output. Domain knowledge and problem-solving skills are crucial in this role, as engineers must navigate both technical challenges and the specific needs of the domain in which they are working. The role is not without its challenges, but the rewards are substantial—prompt engineers are at the forefront of a rapidly evolving field, pushing the boundaries of what AI can achieve. In the next post, we’ll delve deeper into the common challenges faced by prompt engineers and how they can overcome these obstacles to drive innovation in AI